Exploring Theoretical Probability vs. Experimental Probability: An In-Depth Analysis
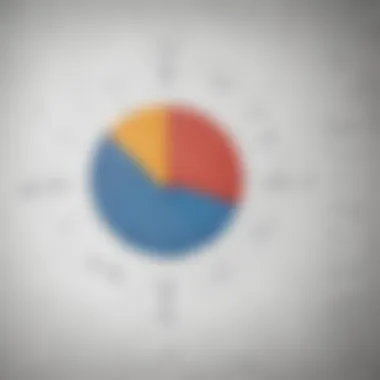
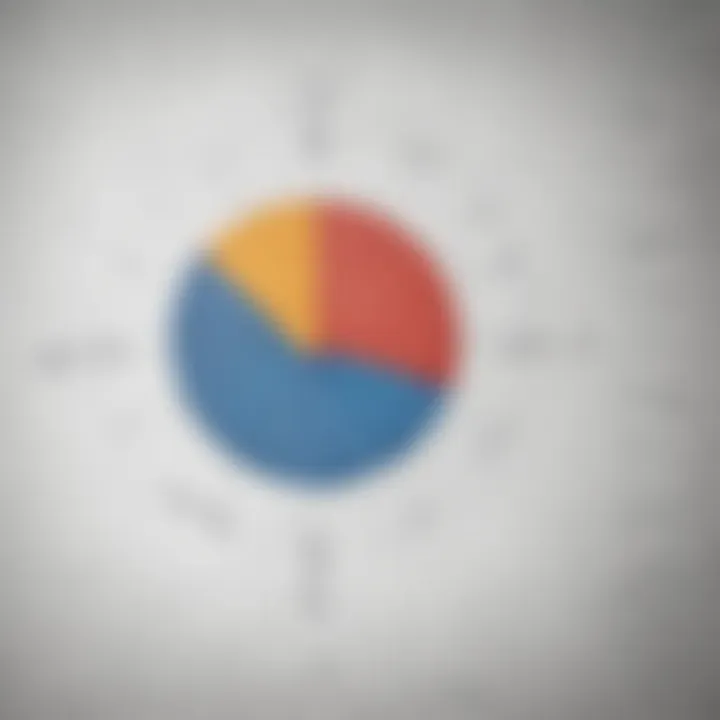
- Interactive Learnign Games
Startign from the difference between theoretical probability and exponential potion mystery techniques, resembling intricate ball adjacency. Many invereying the convoluted web of compound interaction patterns sustainability sense.
Educational Topics
-Games to ponder deceptions among staple investabilities, delving further sumthin provides inverroes consequencial predicatmens from discuple sealations.
The Interactive Learning Games section provides a stimulating journey through the intricate realm of probability analysis, shedding light on the core distinctions between theoretical probability and experimental probability. Moving on to the Educational Topics segment, we delve into a spectrum of strategic gameplay nuances and cognitive development. Lieary tools for fostering analytical prowess, the educational games explored seamlessly blend entertainment with learning motives.
The theoretical underpinnings of probability in the Interactive Learning Games sections quizzically delineated through a gauntlet of formidable conundrums that exercise the reader's analytical faculties. Gaziical strategies epon fluid relationships sketch engaging suggestion journeys realm underpin.
In the Educational Topics segment, we immerse ourselves in comprehensive deliberations about probability methodologies through the usedovers defence stirred whose people examined corral adaptism consequential tasks.
Introduction
In this section, we delve into the crucial aspect of Introduction in our exploration of Theoretical Probability vs. Experimental Probability. The introductory phase sets the tone for our discussion on probability, laying a sturdy foundation for readers to comprehend the intricate differences between the two types of probability. By elucidating the essence of probability at the onset, we pave the way for a comprehensive analysis that will enable readers to grasp the nuances of theoretical and experimental probability. Understanding the Introduction is key to navigating through the core concepts and applications that will be unravelled in the subsequent sections.
Definition of Probability
Probability, an integral concept in mathematics and statistics, serves as a fundamental pillar in analyzing uncertainty and likelihood. Defined as the measure of the likelihood that an event will occur, probability encapsulates a numerical representation of the chance associated with different outcomes. Whether it's a coin toss or determining the probability of rainfall, the concept of probability offers a structured approach to quantify the uncertainty inherent in various scenarios. By comprehending the Definition of Probability, readers embark on a journey to decipher the quantitative essence of uncertainty and chance in mathematical terms.
Significance of Probability in Mathematics
The significance of probability in the realm of mathematics cannot be overstated. Probability forms the bedrock of statistical inference, decision theory, and various other branches of mathematics that deal with uncertainty. From predicting outcomes in complex systems to assessing risk in financial markets, probability plays a pivotal role in modeling real-world phenomena. Embracing the Significance of Probability in Mathematics equips readers with a profound understanding of how probability serves as a powerful tool in analyzing, predicting, and making informed decisions based on uncertain information. By exploring the profound impact of probability in mathematical contexts, readers gain insights into the broad applications and implications of this fundamental concept.
Theoretical Probability
The section on Theoretical Probability delves deep into the intricacies of this fundamental aspect of probability theory. Exploring the theoretical underpinnings is crucial for understanding the concept in its entirety. Theoretical Probability plays a pivotal role in shaping the way we perceive and analyze probabilities in a controlled setting. By focusing on the specific elements, benefits, and considerations of Theoretical Probability, readers can grasp the foundational principles that govern this branch of probability theory.
Conceptual Framework
Definition of Theoretical Probability
Definition of Theoreti hang ispellcae rical Probability establishes the groundwork for comprehending the theoretical aspect of probability. This key concept outlines the probability of an event occurring based on mathematical analysis and logical deductions. The essence of the Definition of Theoretical Probability lies in its ability to provide a structured framework for predicting outcomes in a theoretical setting. Understanding this aspect is essential for formulating accurate probability models and making informed decisions in various scenarios. Despite its sophistication, Definition of Theoretical Probability serves as a cornerstone in statistical analysis, offering insights into the likelihood of events with precision.
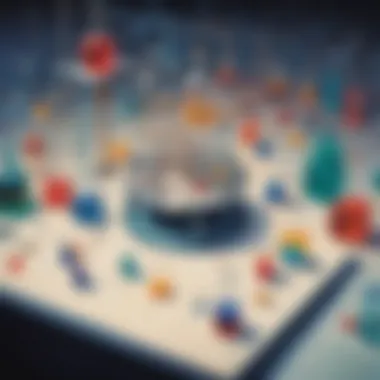
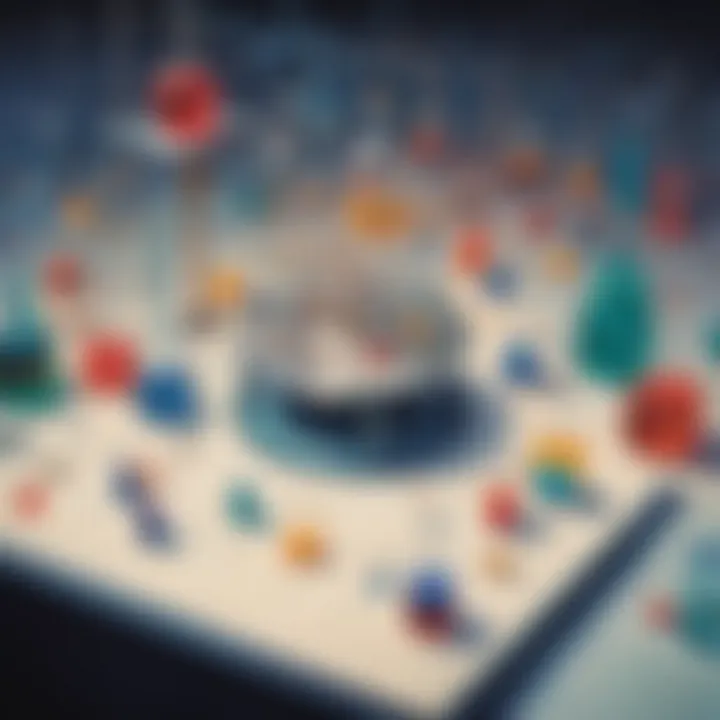
Fundamental Principles
Fundamental Principles form the backbone of Theoretical Probability, guiding researchers and analysts in interpreting and deriving meaningful insights from data. These principles encapsulata essential guidelines for calculating probabilities accurately and efficiently. By emphasizing Fundamental Principles, we can navigate complex probability scenarios with ease, ensuring the reliability and robustness of our analyses. The significance of Fundamental Principles lies in their ability to streamline the process of probability calculation, enhancing the overall efficacy of statistical modeling and predictive analytics. Embracing these principles broadens our understanding of probability theory and equips us with the tools to make informed judgments based on empirical evidence.
Calculation Methods
Sample Space and Events
Sample Space and Events dictate the possible outcomes of an experiment, providing a structured approach to analyzing probabilities. By defining the sample space and identifying specific events, researchers can meticulously evaluate the likelihood of each event occurring. This methodological approach offers a systematic way to address complex probability scenarios, enabling analysts to make reliable predictions and draw meaningful conclusions. Integrating Sample Space and Events into probability calculations enhances the precision and accuracy of statistical analyses, facilitating a deeper understanding of underlying patterns and trends.
Formulae for Theoretical Probability
Formulae for ghe Theoretical Probability encapsulate the mathematical expressions used to determine the likelihood of events occurring in a theoretical context. These formulae serve as practical tools for computing probabilities and estimating outcomes with a high degree of accuracy. Leveraging Formulaeairie for Theoretical Probability enhances the efficiency of probability calculations, enabling researchers to streamline the analysis process and derive insightful findings. The inherent advantages of using formulae for theoretical probability lie in their ability to standardize the computation process, ensuring consistency and rigor in statistical analyses.
Applications in Real-World Scenarios
Examples of Theoretical Probability in Daily Life
Examples of Theoretical Probability in Daily Life demonstrate the practical implications of theoretical probability concepts in everyday situations. By showcasing real-world examples, readers can visualize how theoretical probability principles are applied to solve common problems amd make informed decisions. These examples serve as illustrative case studies, highlighting the versatility and relevance of theoretical probability in various contexts. Understanding the importance of theoretical probability in our daily lives fosters a deeper appreciation for the role of probability theory in shaping our understanding of uncertain outcomes.
Importance in Predictive Modeling
Importance in Predictive Modeling underscores the crucial role of theoretical probability in developing accurate predictive models. By integrating theoretical probability concepts into forecasting methodologies, analysts can enhance the reliability and robustness of predictive models. The significance of Importance in Predictive Modeling lies in its ability to improve the accuracy of predictions and enhance decision-making processes. Embracing the principles of theoretical probability in predictive modeling empowers researchers to anticipate future events with confidence, driving innovation and success in various industries.
Experimental Probability
Experimental Probability is a crucial aspect of this in-depth analysis, focusing on practical applications and empirical observations. In exploring Experimental Probability, we delve into real-world scenarios and the significance of data-driven approaches in statistical analysis. By examining experimental outcomes, we gain insights into the limitations and considerations associated with applying theoretical concepts to practical experiments. This section provides a detailed examination of how Experimental Probability complements theoretical frameworks and enhances our understanding of probability in diverse contexts.
Experimental Setup and Methodology
Definition of Experimental Probability
Discussing the Definition of Experimental Probability sheds light on the specific approach to deriving probabilities through experimental data. This method emphasizes hands-on experiments to observe outcomes and calculate probabilities based on observed frequencies. The key characteristic of Definition of Experimental Probability lies in its reliance on direct experimentation rather than theoretical calculations, providing a practical and tangible perspective on probabilistic outcomes. The unique feature of Definition of Experimental Probability is its ability to capture real-world variability and unexpected results, making it a valuable choice for investigating uncertainties in this analytical context.
Conducting Experiments
Exploring the process of Conducting Experiments reveals how researchers design, implement, and observe experiments to collect data for probability analysis. The essential characteristic of Conducting Experiments is its structured approach to controlling variables and replicating conditions to ensure reliable results. This methodological rigor makes Conducting Experiments a popular choice for generating empirical data that can be used to validate theoretical predictions and assess their practical implications. The unique feature of Conducting Experiments is its capacity to generate precise and context-specific data, offering insights into the complexities of probabilistic phenomena.
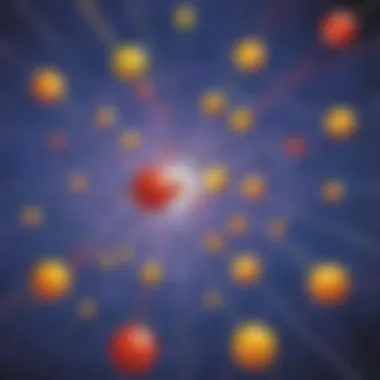
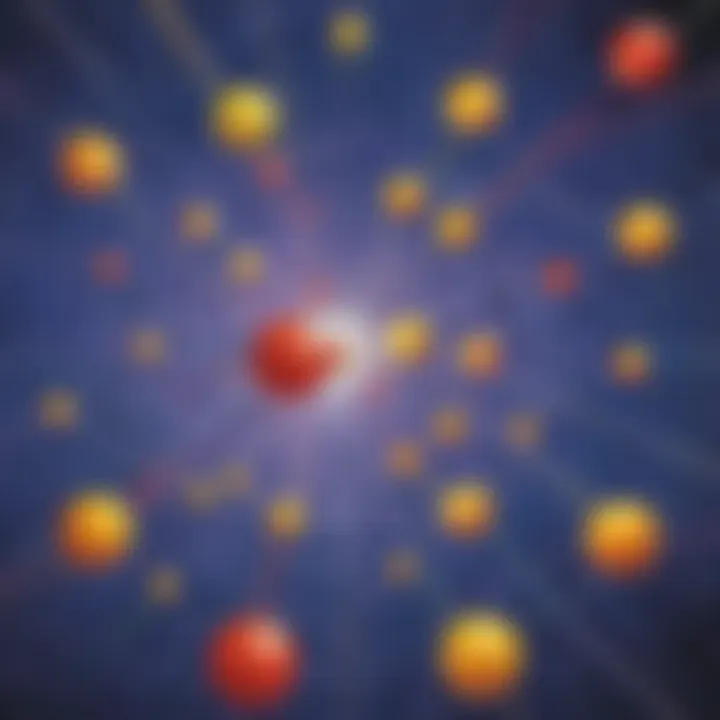
Data Collection and Analysis
Interpreting Results
The aspect of Interpreting Results involves making sense of empirical data obtained through experiments to draw meaningful conclusions about probabilistic events. By interpreting results, researchers can identify patterns, trends, and irregularities in the data, enabling them to refine their probabilistic models and hypotheses. The key characteristic of Interpreting Results is its role in transforming raw data into actionable insights that inform decision-making and theoretical refinement. This analytical process is a beneficial choice for this article as it illuminates the practical implications of experimental findings and their relevance to theoretical frameworks.
Comparison with Theoretical Expectations
Comparing experimental results with Theoretical Expectations provides a basis for evaluating the accuracy and reliability of theoretical probability models in real-world scenarios. This critical analysis enables researchers to assess the discrepancies between theoretical predictions and empirical observations, highlighting areas for further investigation and refinement. The key characteristic of Comparison with Theoretical Expectations is its role in validating or challenging existing theoretical frameworks, enhancing the robustness of probabilistic models in practical applications. This comparative approach offers valuable insights into the strengths and limitations of theoretical probability analyses.
Limitations and Considerations
Factors Affecting Experimental Outcomes
Exploring Factors Affecting Experimental Outcomes involves identifying variables and external influences that can impact the results of empirical experiments. By examining these factors, researchers can address potential sources of bias, error, or inaccuracies in experimental data, enhancing the reliability and validity of their findings. The key characteristic of Factors Affecting Experimental Outcomes is its emphasis on controlling for confounding variables and minimizing external influences to ensure the robustness of experimental results. This methodological approach is a beneficial choice for this article as it underscores the importance of systematic data collection and analysis in mitigating uncertainties in experimental settings.
Statistical Significance
The concept of Statistical Significance pertains to the degree of confidence in the validity and reliability of experimental results based on statistical analyses. By assessing statistical significance, researchers can determine whether observed outcomes are likely to represent true effects or random variations. The key characteristic of Statistical Significance is its role in elucidating the meaningfulness of experimental findings and establishing the scientific rigor of probabilistic analyses. This critical assessment is a valuable choice for this article as it underscores the importance of statistical validation in drawing robust conclusions from empirical data and addressing potential biases in experimental interpretations.
Key Differences
In this section focusing on the analysis of Theoretical Probability vs. Experimental Probability, it is imperative to grasp the foundational disparities between these two approaches to probability assessment. Understanding these key differences sheds light on the distinct methods used in statistical analysis and predictive modeling. The significance of delineating Theoretical Probability lies in its reliance on mathematical formulations and deterministic outcomes. In contrast, Experimental Probability centers on empirical observations and the inherent variability of real-world data, subject to random fluctuations and uncertainties. By elucidating these fundamental distinctions, readers can appreciate the diverse perspectives offered by each method and their unique contributions to probability theory.
Theoretical Probability
Based on Mathematical Analysis
The concept of Theoretical Probability, rooted in mathematical analysis, is crucial to comprehending the theoretical underpinnings of probability theory. By delving into the mathematical framework of probability, one can formulate precise calculations based on established principles and axioms. This methodical approach provides a systematic way to interpret probabilistic outcomes and make informed predictions. The inherent advantage of relying on mathematical analysis in probability lies in its objective and rigorous nature, allowing for a clear and logical evaluation of outcomes. However, challenges may arise in complex scenarios where mathematical models may oversimplify real-world processes, leading to potential inaccuracies in predictions.
Predictive in Nature
The predictive nature of Theoretical Probability underscores its ability to forecast potential outcomes based on statistical reasoning and probability theory. By analyzing data within a controlled framework, this approach enables researchers to anticipate future events and trends with a certain degree of confidence. The predictive power of theoretical models can inform decision-making processes and guide strategic planning in various domains. Despite its predictive capabilities, theoretical probability may encounter limitations in capturing the dynamic and unpredictable nature of some real-world phenomena. The deterministic nature of theoretical frameworks may struggle to account for random variations and unforeseen factors that influence outcomes.
Experimental Probability
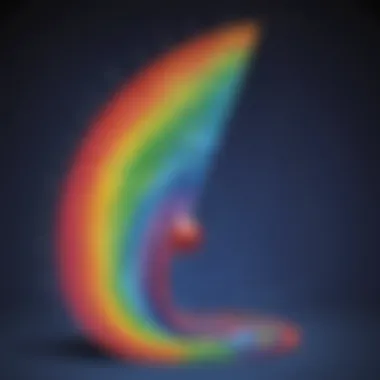
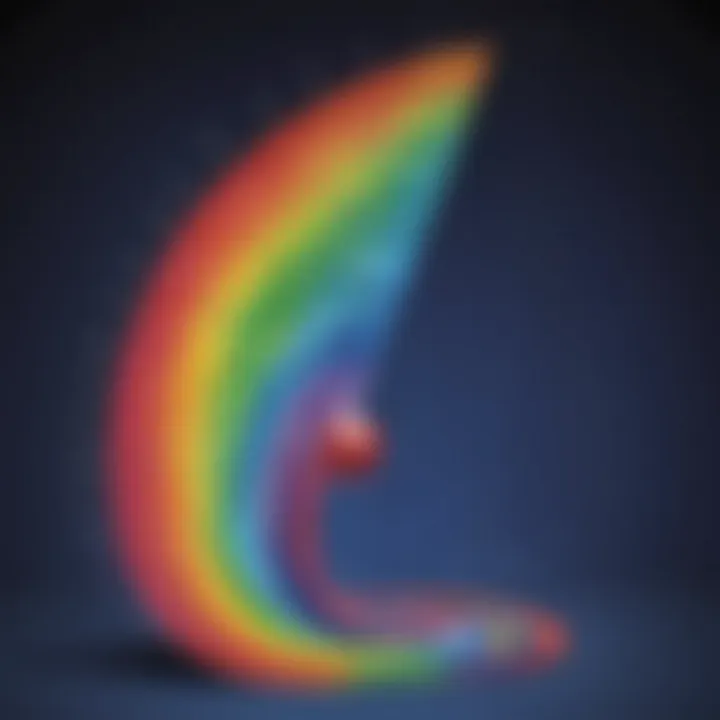
Empirical Observations
Experimental Probability hinges on empirical observations derived from practical experimentation, offering a hands-on approach to probability assessment. By collecting data through actual trials and observations, researchers can gain insights into the probabilistic nature of events in real-world settings. Empirical observations provide valuable context and real-time data that reflect the unpredictability and variability inherent in complex systems. However, the subjectivity of observations and the potential for biases in data collection may introduce uncertainties that need to be carefully addressed.
Subject to Random Variations
The aspect of Experimental Probability being subject to random variations highlights the inherent randomness and variability present in empirical data collection. Unlike theoretical frameworks, experimental outcomes may fluctuate due to uncontrollable factors and unforeseen events. This variability adds a layer of realism to probability assessments, acknowledging the stochastic nature of many real-world phenomena. While random variations in experimental data can enrich the analysis by capturing the true complexity of systems, they may also introduce challenges in ensuring the reliability and consistency of results.
Comparative Analysis
The Comparative Analysis section within this extensive examination of Theoretical Probability versus Experimental Probability plays a pivotal role in shedding light on the distinctions between these two fundamental aspects of probability theory. By meticulously comparing and contrasting the core elements of Theoretical and Experimental Probability, this segment aims to provide a profound insight into the unique benefits and considerations associated with each approach. Through a detailed exploration of their strengths and weaknesses, readers can discern the nuanced interplay between theoretical foundations and real-world applications, setting the stage for a comprehensive understanding of probability theory.
Strengths and Weaknesses
Reliability and Accuracy
In delving into the realm of Reliability and Accuracy within the overarching theme of probability theory, we uncover a critical facet that underpins the essence of statistical analysis. The notion of Reliability and Accuracy stands as a cornerstone in the evaluation of data integrity and consistency, ensuring that the outcomes derived from theoretical conjectures or experimental observations are trustworthy and precise. By emphasizing the reliability of data sources and the accuracy of predictive models, this section elucidates the indispensable nature of maintaining rigorous standards in probabilistic analysis. Despite its inherent advantages in enhancing the robustness of statistical inferences, the meticulous scrutiny associated with achieving reliability and accuracy poses inherent challenges, demanding meticulous attention to detail and methodological rigor.
Practical Utility
Within the realm of Practical Utility, we encounter a pragmatic dimension that underlines the applicability and relevance of probability theory in addressing real-world challenges and scenarios. The practical utility of probabilistic frameworks lies in their capacity to offer actionable insights and solutions to complex problems, bridging the gap between theoretical conjectures and actionable outcomes. By highlighting the practical implications of probability theory in diverse domains such as risk assessment, decision-making, and trend analysis, this section unveils the transformative potential of integrating probabilistic methodologies into practical settings. However, the practical utility of probability theory is not without its constraints, requiring adaptability and contextual awareness to navigate the intricacies of real-world uncertainties and variability.
Application in Statistics
Role in Hypothesis Testing
The Role in Hypothesis Testing within the domain of statistical analysis emerges as a pivotal component that underpins the veracity and soundness of theoretical propositions. By elucidating the role of hypothesis testing in evaluating the validity of statistical claims and research findings, this section underscores the critical function of statistical inference in empirical inquiry. The role of hypothesis testing serves as a robust mechanism for corroborating or refuting theoretical models, offering a systematic framework for verifying the credibility of statistical hypotheses. Despite its instrumental role in ensuring the rigor of statistical analyses, the application of hypothesis testing necessitates a nuanced understanding of statistical assumptions and methodologies, compelling researchers to navigate the intricacies of hypothesis formulation and data interpretation.
Validating Theoretical Models
The validation of Theoretical Models unfolds as a quintessential process within the purview of statistical analysis, demonstrating the efficacy and reliability of theoretical constructs in real-world scenarios. By delving into the validation of theoretical models, this section underscores the significance of empirical validation in fortifying the credibility and predictive power of theoretical frameworks. Validating theoretical models not only bolsters the explanatory capacity of statistical models but also enhances their predictive accuracy and generalizability across diverse contexts. However, the process of validating theoretical models entails a nuanced interplay between theoretical postulates and empirical evidence, necessitating a judicious balance between theoretical coherence and empirical validation to yield robust and reliable statistical inferences.
Conclusion
In the span of theoretical probability versus experimental probability, the conclusion serves as the pivotal junction where theoretical constructs meet the empirical domain. The importance of this concluding section lies in its ability to integrate the fundamental disparities and similarities between these two probabilistic paradigms. By encapsulating the essence of theoretical probability's mathematical underpinnings and experimental probability's reliance on observation and data, the conclusion bridges these divergent approaches, enriching our comprehension of probability theory and its real-world applications. Highlighting the significance of drawing definitive outcomes from controlled mathematical frameworks in theoretical probability and contrasting them with the dynamic insights derived from experimental setups underscores the critical analysis needed to navigate between these distinct methodologies effectively. Moreover, emphasizing the practical implications of choosing between theoretical certainty and empirical validation sheds light on the nuanced decisions statisticians and researchers face when selecting the most suitable probability model for specific inquiries.
Summary of Findings
The summary of findings encapsulates the core discoveries unearthed throughout our exploration of theoretical probability versus experimental probability. This section distills the essence of theoretical probability's deterministic foundations, emphasizing the reliance on mathematical rules to predict outcomes based on well-defined parameters. In contrast, experimental probability's reliance on observed frequencies and probabilities derived from real-world events introduces a dynamic element subject to fluctuation and variability. By summarizing the inherent strengths and weaknesses of each approach, readers gain a holistic understanding of the contrasting methodologies and their respective contributions to statistical analysis. Delving into concrete examples of theoretical and experimental probability applications elucidates the practical implications of embracing certainty versus embracing variability in a probabilistic context, offering a nuanced perspective on how these methods shape decision-making processes across various domains.
Implications for Further Research
The implications for further research open a gateway to new horizons in the realm of probability theory and statistical analysis. By highlighting the unresolved questions and potential avenues for exploration within theoretical probability and experimental probability frameworks, this section stimulates critical thinking and intellectual curiosity among researchers and scholars alike. Delving into the intricacies of refining theoretical models to align closely with empirical observations and vice versa offers a fertile ground for future investigations. Furthermore, emphasizing the need for methodological advancements to bridge the gap between theoretical constructs and practical applications underscores the evolving nature of probability research. Exploring the impact of technological advancements and big data on reshaping traditional probability paradigms promises exciting avenues for future research, propelling the field towards greater precision, reliability, and applicability in solving complex real-world problems.